PUBLICATIONs
My research areas are in Semiconductor Manufacturing, Smart Manufacturing, Industry 4.0, Deep Learning, Quantum Computing, Technology Art, and Environment Art. I conduct research and production across fields such as defect inspection and yield prediction using deep learning techniques. In TechArt, I have studied the use of artificial intelligence (AI) to enhance human creativity. My artworks were selected for the 2021 Ars Electronica Festival.
Semiconductor Defect Detection by Hybrid Classical-Quantum Deep Learning
CVPR'22
# Quantum Computing
In this paper, we utilize the information processing advantages of quantum computing to promote the defect learning defect review (DLDR). We propose a classical-quantum hybrid algorithm for deep learning on near-term quantum processors. By tuning parameters implemented on it, quantum circuit driven by our framework learns a given DLDR task, include of wafer defect map classification, defect pattern classification, and hotspot detection. In addition, we explore parametrized quantum circuits with different expressibility and entangling capacities. These results can be used to build a future roadmap to develop circuit-based quantum deep learning for semiconductor defect detection.
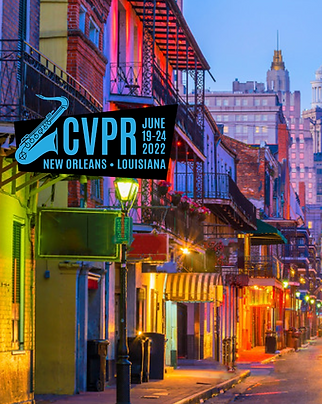
Hybrid Quantum-Classical Machine Learning for Lithography Hotspot Detection
ASMC'22
# Quantum Computing
In advanced semiconductor process technology, lithography hotspot detection has become an essential task in design for manufacturability. The ability to detect and repair lithography hotspots that can affect printability is critical to improving yield and productivity. We explore the possibility of converting a novel machine learning model to a hybrid quantum-classical machine learning that benefits from using variational quantum layers. We show that this hybrid model can perform similar to the classical approach. In addition, we explore parametrized quantum circuits (PQC) with different expressibility and entangling capacities. Then we compare their training performance to quantify the expected benefits.

Medium . Permeation
Ars Electronica 2021
# TechArt
Impressionism expressed the scientific meaning of "light" in artistic creation. The light color reflected by the luminous flux through the medium in the air became the concept of impressionists’ creation. After collecting the web imagination of global citizens about COVID_19, we trained the generative model and let the computer randomly synthesize it. We adjusted various parameters during the training process making the results such as Dou Jia, Monet, Renoir , their style paintings. Through this work the diffusion path of COVID_19 spreading the molecular of the virus through the air, just like the scattering phenomenon formed by light through the particles in the air. Color is exclusive to the humans’ world, and COVID_19 is also born with humans. The colorful world we see in this work represents the equality of races on the earth. The array of 196 pictures represents 196 countries were invaded by the COVID_19. As human beings, none of us are spared from this disaster.
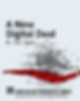
Semiconductor Defect Pattern Classification by Self-Proliferation-and-Attention Neural Network
IEEE Transactions on Semiconductor Manufacturing
# Deep Learning, Semiconductor Manufacturing, Industry 4.0
In this paper, we present a novel architecture that can perform defect classification in a more efficient way. The first function is self-proliferation, using a series of linear transformations to generate more feature maps at a cheaper cost. The second function is self-attention, capturing the long-range dependencies of feature map by the channel-wise and spatial-wise attention mechanism. We named this method as self-proliferation-and-attention neural network (SP&A-Net). This method has been successfully applied to various defect pattern classification tasks. Compared with other latest methods, SP&A-Net has higher accuracy and lower computation cost in many defect inspection tasks.
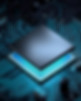
A Novel Deep Learning Architecture for Global Defect Classification: Self-Proliferating Neural Network
2021 32nd Annual SEMI Advanced Semiconductor Manufacturing Conference (ASMC)
# Deep Learning, Semiconductor Manufacturing, Industry 4.0
We have developed a new deep learning architecture, named Self-Proliferating Neural Network (SPNet). Compared with other methods, SPNet can significantly reduce false positives and false positives, while improving quality and productivity. We also show that our method generalizes well to other public datasets, where they achieve state-of-the-art results. Finally, we apply SPNet to the classification tasks of defect map and defect pattern, and the F1-score achieves 98.9% and 98.2%, respectively. We conduct experiments that probe the robustness of learned representations and conclude that SPNet has significant benefits in robustness and generalization.

Double Feature Extraction Method for Wafer Map Classification Based on Convolution Neural Network
2020 31nd Annual SEMI Advanced Semiconductor Manufacturing Conference (ASMC)
Best Student Paper Award in ASMC'20
# Deep Learning, Semiconductor Manufacturing
In this paper, we propose double feature extraction method based on convolution neural network. The proposed model uses the Radon transform for the first feature extraction, and then input this feature into the convolution layer for the second feature extraction. Experiments with real-world data set verified that the proposed method achieves high defect classification performance, defect pattern recognition accuracy up to 98.5%, and we confirmed the effectiveness of the proposed feature extraction technique.

A Deep Learning Model for Identification of Defect Patterns in Semiconductor Wafer Map
2019 30nd Annual SEMI Advanced Semiconductor Manufacturing Conference (ASMC)
# Deep Learning, Semiconductor Manufacturing
we employ convolutional neural networks (CNN) and extreme gradient boosting (XGBoost) for wafer map retrieval tasks and the defect pattern classification. CNN and XGBoost are compared with a random decision forests (RF), support vector machine (SVM), adaptive boosting (Adaboost), and the final results indicate a superior classification performance of the proposed method. Our experimental result demonstrates the success of CNN and extreme gradient boosting techniques for the identification of defect patterns in semiconductor wafers. The overall classification accuracy for the test dataset of CNN and extreme gradient boosting is 99.2%/98.1%. We demonstrate the success of this technique for the identification of defect patterns in semiconductor wafers. We believe this is the first time accurate computational classification in such task has been reported achieving accuracy above 99%.
